The Importance of Data Labeling for Modern Businesses
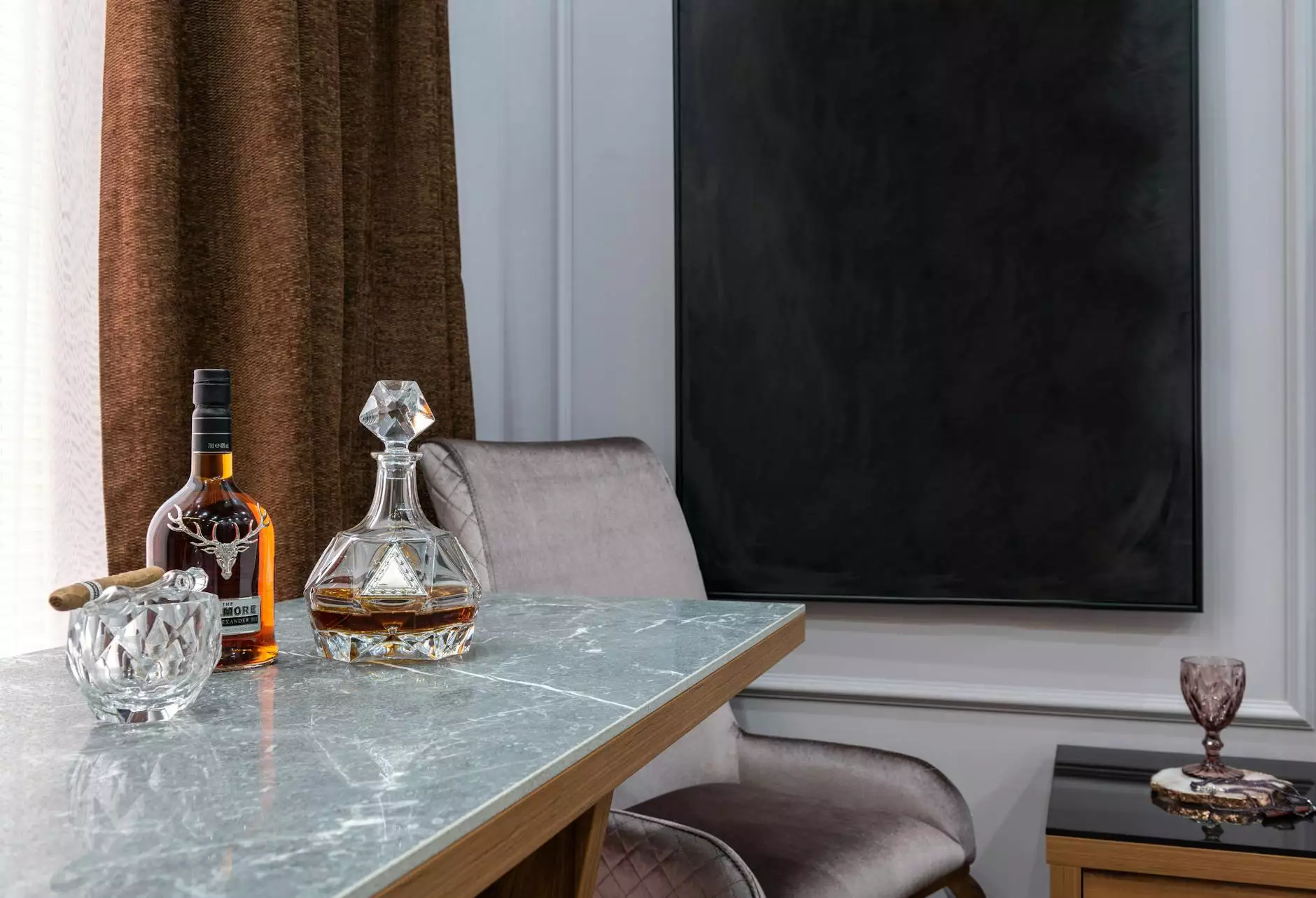
In the digital era, data has become the lifeblood of businesses across all sectors, from home services to specialized industries like keys and locksmiths. As companies strive for *enhanced efficiency* and *better decision-making*, data labeling emerges as a critical component in managing and utilizing these vast amounts of information effectively.
What is Data Labeling?
Data labeling refers to the process of annotating or tagging data with relevant labels that can be used for training machine learning models. This process allows algorithms to learn from the input data and identify patterns, making it essential for applications in artificial intelligence (AI) and machine learning (ML).
Why is Data Labeling Vital for Businesses?
The significance of data labeling cannot be overstated, as it plays a pivotal role in various business functions. Let's explore its core benefits:
1. Enhanced Data Accuracy
Accurate data is crucial for any organization. With data labeling, businesses can ensure that their datasets are reliable and well-understood. Accurate labels lead to better model training and improved predictive accuracy, which are vital for making informed business decisions.
2. Improved Customer Insights
For companies operating in home services or locksmithing, understanding customer behavior is paramount. Data labeling helps in segmenting customer data based on various attributes, enabling businesses to tailor their marketing strategies effectively and target the right audience.
3. Accelerated Machine Learning Development
With the increasing reliance on AI and ML, companies require labeled data to train their models efficiently. The speed and quality of model development can be significantly enhanced through effective data labeling practices, allowing businesses to stay ahead in a competitive landscape.
How Data Labeling Works
The data labeling process typically involves several key steps:
- Data Collection: The first step is gathering raw data, which can come from various sources like user interactions, sensor data, or files.
- Data Annotation: Once the data is collected, it must be annotated by human labelers or automated tools. This involves adding relevant labels, categories, or tags to the data.
- Quality Assurance: Ensuring quality is critical. Businesses need to verify the accuracy of the labels through rigorous testing methods, which may include double-checking and peer reviews.
- Data Utilization: The labeled data is then used for training machine learning models, which help in automating and improving various business functions.
Applications of Data Labeling Across Industries
Data labeling is not confined to a single sector; its applications are extensive and varied. Here are some key areas where data labeling makes a significant impact:
1. Healthcare
In the healthcare sector, data labeling is critical for training AI systems that assist in diagnoses, treatments, and patient care. Labeled medical images, for instance, can help in detecting diseases with remarkable accuracy.
2. Retail and E-Commerce
Retail businesses can leverage data labeling to enhance customer experience by analyzing shopping behaviors, preferences, and engagement. This insights-driven approach leads to personalized recommendations and improved customer satisfaction.
3. Autonomous Vehicles
The automotive industry, especially those venturing into autonomous driving, relies heavily on labeled data for training self-driving car systems. Labeled images and sensor data enable vehicles to interpret their surroundings accurately, ensuring higher safety standards.
4. Social Media Management
Social media platforms utilize data labeling to analyze sentiments, categorize content, and improve user engagement strategies. Through data labeling, brands can analyze customer feedback effectively and respond promptly.
Benefits of Outsourcing Data Labeling Services
While in-house data labeling can be beneficial, outsourcing to specialized data labeling service providers can offer several advantages:
- Cost-Effectiveness: Outsourcing can significantly reduce operational costs associated with hiring and training in-house teams.
- Scalability: External providers can offer flexible solutions that grow with your business needs, accommodating varying volumes of data.
- Expertise: Specialized firms bring in-depth experience and better tools to ensure high-quality data labeling with minimal errors.
- Focus on Core Activities: Outsourcing allows businesses to focus on their core functions while leaving the intricacies of data labeling to experts.
Challenges in Data Labeling Efforts
Despite its advantages, data labeling also faces certain challenges:
1. Quality Concerns
Maintaining high labeling quality consistently is challenging. Inaccurate or inconsistent labeling can lead to poor model performance, which can adversely affect business outcomes.
2. Time-Intensiveness
The data labeling process can be labor-intensive and time-consuming, particularly when dealing with vast datasets. This can delay the overall project timeline.
3. Data Privacy Issues
Handling sensitive data requires strict adherence to privacy regulations. Businesses must ensure that their labeling practices comply with legal standards to avoid any potential breaches.
Future of Data Labeling in Business
The future of data labeling looks promising, with advancements in technology continuously evolving the landscape. Here are some trends to keep an eye on:
1. Automation
With advancements in machine learning and AI, data labeling processes are becoming increasingly automated. Reducing manual intervention not only speeds up the process but also enhances accuracy over time.
2. Focus on Ethics
As businesses become more aware of ethical considerations around data use, responsible data labeling that respects privacy and inclusivity will gain prominence.
3. Growth of Synthetic Data
The rise of synthetic data—data generated from algorithms rather than collected from real-world sources—will impact data labeling. Such data will require innovative labeling techniques that differ from traditional methods.
Conclusion
In summary, data labeling is an indispensable part of the modern data landscape, crucial for businesses looking to leverage data for smarter decision-making. From home services to keys and locksmiths, firms need to prioritize quality labeling practices to ensure their AI and ML initiatives are successful. As technology evolves, adopting effective data labeling strategies will allow businesses to gain a competitive edge, enhance operational efficiency, and ultimately provide better value to their customers.
Understanding the importance of data labeling and its multitude of applications can empower businesses to harness their data and turn challenges into opportunities. Now is the time to invest in establishing sound data labeling processes that will pave the way toward future success.